일 | 월 | 화 | 수 | 목 | 금 | 토 |
---|---|---|---|---|---|---|
1 | 2 | 3 | ||||
4 | 5 | 6 | 7 | 8 | 9 | 10 |
11 | 12 | 13 | 14 | 15 | 16 | 17 |
18 | 19 | 20 | 21 | 22 | 23 | 24 |
25 | 26 | 27 | 28 | 29 | 30 | 31 |
- 머신러닝
- databasemanagementsystem
- MachineLearning
- human-interface-guide
- ML
- database
- lightweightproces
- threeschemaarchitecture
- OperatingSystem
- xv6
- 자료구조
- mlfq
- db
- softmargin
- dbms
- monopolyqueue
- dataindependency
- SVM
- 기계학습
- 운영체제
- copyonwrite
- databasesystems
- conceptualdatamodeling
- entity-relationshipmodel
- softmarginsvm
- SupportVectorMachine
- react native #rn #리액트네이티브 #hook #hooks #훅 #navigation #네비게이션 #usenavigate
- humaninterfaceguide
- Datastructure
- multilevelfeedbackqueue
- Today
- Total
목록기계학습 (4)
leehyogum의 트러블슈팅
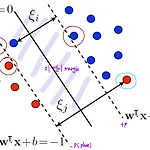
이전 포스팅까지는 Hard margin SVM에 대해 설명하였다. [Machine Learning] Support Vector Machine (SVM) (3)이전 글까지는 Hard margin SVM의 optimization problem의 primal form을 유도하였다.이번 글에서는 Hard margin SVM의 최종 problem인 dual problem 및 최적화 과정까지 유도해보도록 하겠다. [Machine Learning] Support Vectorleehyogum.tistory.com 이번 포스트에서는 Soft margin SVM에 대해 설명하겠다. 이전 포스트에서까지 언급했듯, Hard margin SVM은 linearly separable한 데이터에 대해서만 사용할 수 있다.그럼 li..
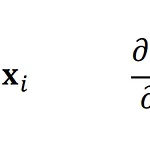
이전 글까지는 Hard margin SVM의 optimization problem의 primal form을 유도하였다.이번 글에서는 Hard margin SVM의 최종 problem인 dual problem 및 최적화 과정까지 유도해보도록 하겠다. [Machine Learning] Support Vector Machine (SVM) (2)이전 글에서 SVM에 대한 기본적인 개념을 설명하였다. [Machine Learning] Support Vector Machine (SVM) (1)우리에게 Leanearly separable 한 두 개의 클래스 중 하나의 클래스에 속하는 데이터셋들이 있다.우리의leehyogum.tistory.com Lagrange Multipler를 이용하여 Hard SVM을 푸는 ..
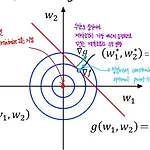
이전 글에서 SVM에 대한 기본적인 개념을 설명하였다. [Machine Learning] Support Vector Machine (SVM) (1)우리에게 Leanearly separable 한 두 개의 클래스 중 하나의 클래스에 속하는 데이터셋들이 있다.우리의 데이터셋을 두 개의 class로 구분하는 decision boundary A와 B 중 어느 것이 더 좋은 decision boundary일leehyogum.tistory.com 이번 포스트에서는 SVM의 optimization problem을 푸는 방법에 대해 설명해보도록 하겠다. SVM의 object function은 다음과 같다. min $\frac{1}{2} ||w^2||$subject to $y_i(w^TX_i + b) ≥ 1$, ∀$i..
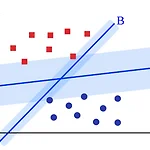
우리에게 Leanearly separable 한 두 개의 클래스 중 하나의 클래스에 속하는 데이터셋들이 있다.우리의 데이터셋을 두 개의 class로 구분하는 decision boundary A와 B 중 어느 것이 더 좋은 decision boundary일까?대충 감으로도 알 수 있듯 정답은 바로 A이다. 그 이유는 A가 B보다 일반적인 성능이 더 좋기 때문이다.주어진 test data들에 대한 성능만 보는 것이 아니라, 미래에 들어올 data도 잘 분류할 가능성이 높은 A가 B보다 좋다. 다시 말해서, A가 B보다 각 클래스 데이터에 대해 높은 margin을 주는 분류기이기 때문이다. Margin이란?Margin이란, decision boundary와 decision boundary에서 가장 가까운 da..